Can AI Reason? And Why It Matters for Ethics
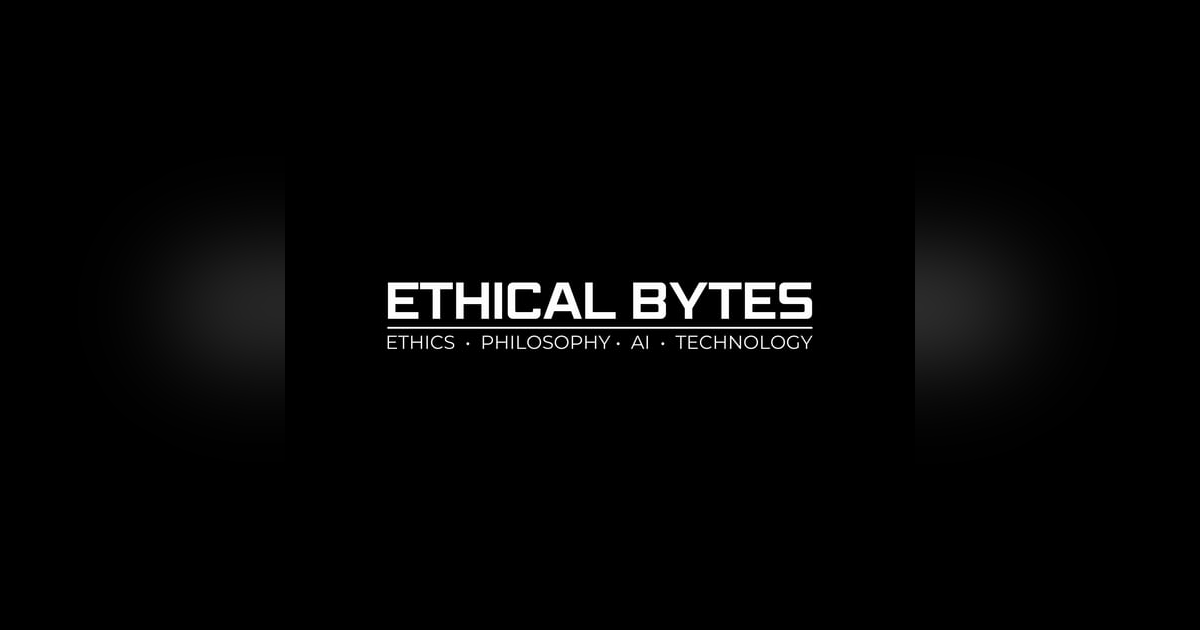
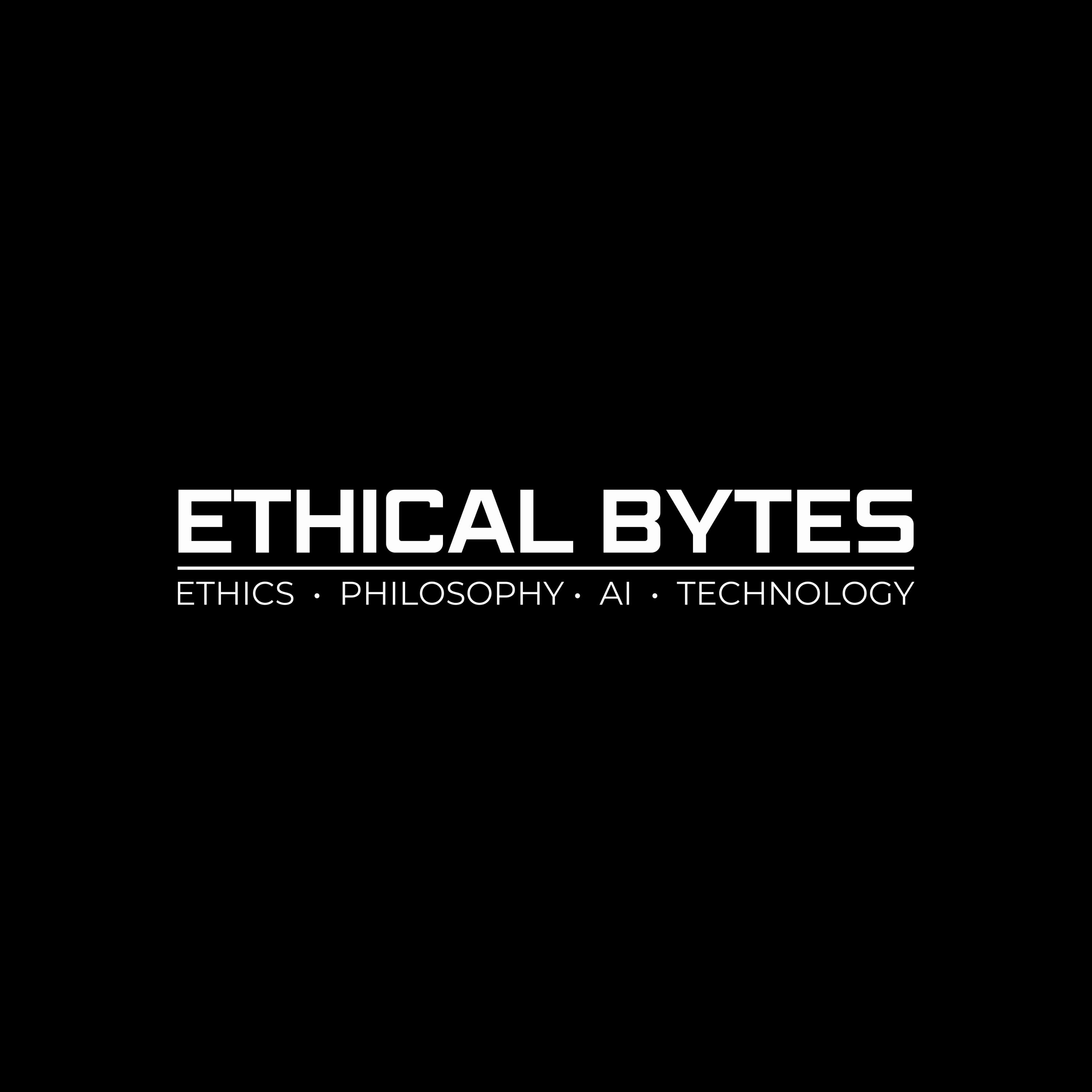
Will AI’s ever-evolving reasoning capabilities ever align with human values?
Day by day, AI continues to prove its worth as an integral part of decision-making, content creation, and problem-solving. Because of that, we’re now faced with the question of whether AI can truly understand the world it interacts with, or if it is simply doing a convincing job at identifying and copying patterns in human behavior. Our host, Carter Considine, breaks it down in this episode of Ethical Bytes.
Indeed, some argue that AI could develop internal "world models" that enable it to reason similarly to humans, while others suggest that AI remains a sophisticated mimic of language with no true comprehension.
Melanie Mitchell, a leading AI researcher, discusses the limitations of early AI systems, which often relied on surface-level shortcuts instead of understanding cause and effect. This problem is still relevant today with large language models (LLMs), despite claims from figures like OpenAI’s Ilya Sutskever that these models learn compressed, abstract representations of the world.
Then there are critics, such as Meta's Yann LeCun, who argue that AI still lacks true causal understanding–a key component of human reasoning–and thus can never make true ethical decisions.
Advancements in AI reasoning such as "chain-of-thought" (CoT) prompting improves LLMs’ ability to solve complex problems by guiding them through logical steps. While CoT can help AI produce more reliable results, it doesn't necessarily mean the AI is “reasoning” in a human-like way—it may still just be an advanced form of pattern matching.
Clearly, as AI systems become more capable, the ethical challenges multiply. AI's potential to make decisions based on inferred causal relationships raises questions about accountability, especially when its actions align poorly with human values.
Key Topics:
- Can AI Reason? (00:00)
- Pattern-Matching vs. Understanding (01:14)
- Does AI Understand Cause and Effect? (05:44)
- Chain-of-Thought Prompting (06:52)
- Ethical Implications of AI Reasoning (09:32)
- Wrap-Up (12:08)
More info, transcripts, and references can be found at ethical.fm
AI has become an integral part of decision-making processes, content creation, and problem-solving tasks that have been traditionally reserved for humans. As these systems have grown in complexity, a fundamental question keeps emerging: does AI actually understand the world it interacts with, or is it simply an advanced pattern-matching system? Although heavily debated in academia, the discussion moves beyond the ivory tower and has profound implications for practical applications and AI ethics. If AI systems form internal "world models," their outputs may reflect forms of reasoning similar to human understanding. If not, they remain sophisticated parrots, mimicking language without comprehension.
Pattern-Matching vs. Understanding
Understanding how AI processes information, especially its ability (or lack thereof) to grasp cause and effect, is essential for evaluating these opposing views. Melanie Mitchell’s Substack series, LLMs and World Models, highlights the core of this debate. Mitchell is a professor of computer science at the Santa Fe Institute specializing in AI, cognitive science, and complex systems. She is known for her work on analogical reasoning in AI, computational models of intelligence, and the broader implications of AI on society.
In early AI systems, brittleness was a known problem. Brittleness refers to AI models learning “shortcuts” or “surface heuristics” that are specific to its training data rather than abstract, causal understanding as human trainers were trying to teach it. For instance, a medical image classifier misinterpreted the presence of rulers in photos as a sign of malignancy, “[T]he algorithm appeared more likely to interpret images with rulers as malignant. Why? In our dataset, images with rulers were more likely to be malignant; thus, the algorithm inadvertently ‘learned’ that rulers are malignant.” These heuristic “shortcuts” are found across all types of data, including in text.
With LLMs, huge neural networks pre-trained on enormous amounts of human-created text data, the conversation has become more complex. LLMs seem to perform much better than the previous paradigm of machine learning systems. For instance, OpenAI's recent model, o1, claims to possess enhanced reasoning capabilities, suggesting the development of an internal “world model.” Ilya Sutskever, cofounder of OpenAI, claims, “When we train a large neural network to accurately predict the next word in lots of different texts...it is learning a world model.... This text is actually a projection of the world... What the neural network is learning is more and more aspects of the world, of people, of the human conditions, their hopes, dreams, and motivations...the neural network learns a compressed, abstract, usable representation of that.”
But what exactly is a world model? There are several definitions from academia, such as “[I]nternal representations that simulate aspects of the external world” and “[R]epresentations which preserve the causal structure of the environment as far as is necessitated by the tasks an agent needs to perform.” According to Mitchell, these definitions emphasize that world models exist in the mind of an organism, or, analogously, in an LLM’s neural network. These world models capture parts of reality that contain causal and abstract (or compressed) information. Mitchell describes a world model as an internal representation of how the world works, allowing an agent to predict outcomes and reason about unseen situations. For humans, this is what enables us to navigate new environments with ease. For example, if someone has never been to an airport before but understands general concepts like security checkpoints, boarding gates, and baggage claims, they can still successfully move through the airport by applying their prior knowledge and reasoning about what to do next.
Critics argue that without true causal understanding, AI models might still be performing advanced pattern matching by memorizing its training data and retrieving it in an “approximate” way. This is the view of many prominent AI researchers, such as Meta’s Yann LeCun. LeCun, along with philosopher Jacob Browning, assert that “A system trained on language alone will never approximate human intelligence, even if trained from now until the heat death of the universe.”
Does AI understand cause and effect?
Understanding causality is a cornerstone of human reasoning. Humans can distinguish between correlation and causation, a skill that underpins ethical decision-making. If AI systems cannot grasp cause and effect, their outputs could inadvertently reinforce flawed assumptions or make decisions that conflict with human values without the capacity for correction.
In the article Artificial Intelligence Is Stupid and Causal Reasoning Will Not Fix It, Mark Bishop argues that while AI can identify patterns, models inherently lack the ability to understand causal relationships. This limitation suggests that without integrating causal models in some form, AI may continue to make errors in judgment that have ethical consequences.
However, advancements are being made. The World Models: The Safety Perspective paper discusses how AI systems can develop internal representations that mirror the causal structures of the world, potentially leading to more reliable and ethically sound decision-making processes.
Chain-of-Thought (CoT) Prompting
A pivotal advancement in AI reasoning capabilities is the introduction of chain-of-thought (CoT) prompting. According to the paper Chain-of-Thought Prompting Elicits Reasoning in Large Language Models, CoT prompting significantly enhances LLMs' ability to solve complex reasoning tasks by guiding them to break down problems into intermediate steps.
How Chain-of-Thought Prompting Works
Chain-of-thought prompting works by structuring AI responses in a way that mimics human reasoning. Instead of directly producing an answer, the model is guided to explicitly articulate each step leading to the final conclusion. For example, when solving a math word problem, the AI first breaks down the numerical relationships, calculates intermediary results, and then combines them to reach the final answer. This structured approach helps the model improve accuracy, especially on problems requiring multiple reasoning steps. Here’s a simple example:
Standard Prompting:
- Question: A store had 20 apples. It sold 8 and then received a shipment of 5 more. How many apples does the store have now?
- AI Answer: 17
Chain-of-Thought Prompting:
- Question: A store had 20 apples. It sold 8 and then received a shipment of 5 more. How many apples does the store have now?
- AI Answer: The store started with 20 apples. After selling 8, it had 20 - 8 = 12 apples. Then, it received 5 more apples, bringing the total to 12 + 5 = 17 apples. The final answer is 17.
By explicitly working through each step, the AI demonstrates a form of structured reasoning that is more reliable and interpretable. This method has been particularly effective in arithmetic, commonsense reasoning, and symbolic reasoning tasks.
But does this mean AI is reasoning in a human-like way? Not necessarily. CoT prompting could simply still be a more sophisticated form of pattern matching, optimized to align with human problem-solving structures. However, the fact that LLMs can be guided to emulate causal reasoning pathways is significant. It blurs the line between mere pattern retrieval and genuine understanding.
Ethical Implications of AI Reasoning
The distinction between pattern matching and world modeling has real-world ethical implications. If LLMs develop world models, even implicitly, they could be seen as agents capable of flawed reasoning. This presents a complex challenge in determining accountability when AI systems make harmful or misguided decisions. While AI lacks consciousness or intent, its ability to produce outputs based on inferred causal relationships complicates the ethical landscape. It challenges the simplicity of holding only human developers accountable when an AI system causes harm.
Another significant ethical concern lies in the fact that all data used to train AI systems carries inherent normative assumptions, or implicit ideas about what matters, what is valuable, and how decisions should be made. These assumptions shape the behavior of AI models in ways that are difficult to identify but deeply consequential. Importantly, these embedded norms cannot be entirely removed or neutralized; they are intrinsic to the data itself. Ethical decision-making, by definition, involves the application of moral principles to specific situations, weighing competing values, and considering not just outcomes but intentions, contexts, and duties. AI systems, trained on data reflecting human perspectives, inevitably inherit these layered and sometimes conflicting values.
Whether made apparent or not, the ethical assumptions within AI models influence the kinds of actions a model may take in complex social environments. If an AI system mimics causal reasoning without truly understanding the implications of its actions, its deployment in scenarios requiring nuanced judgment may lead to a considerable number of unintended consequences. Techniques like CoT make the path to understanding AI behavior more convoluted. Training data will not be enough to predict AI behavior, since there are many decisions CoT models make over time. Controlling all model outputs will become difficult since there will be many opportunities for potential misbehavior, making building ethical machines even more complex. Similarly to teaching a model causal reasoning, developers will need to teach AI systems human moral reasoning as well.
Conclusion
The ongoing debate about AI’s capacity for causal reasoning and world modeling is central to ethical AI development. If LLMs are more than just stochastic parrots, forming abstract representations and reasoning about cause and effect, then they carry a weight of responsibility previously reserved for human agents.
Chain-of-thought prompting exemplifies the blurred boundary between pattern matching and reasoning. While it enables AI to tackle more complex tasks, it also introduces new ethical complexities. Understanding how AI processes information isn’t just a technical concern; it’s foundational to creating systems that align with human values and societal norms.
As AI continues to evolve, grappling with these questions will determine how responsibly we integrate these systems into our world. The stakes are high, not just for developers and ethicists, but for everyone living in a world increasingly shaped by artificial intelligence.