The Price of Precision: Data Labeling and the Debate Over ‘Digital Sweatshops’
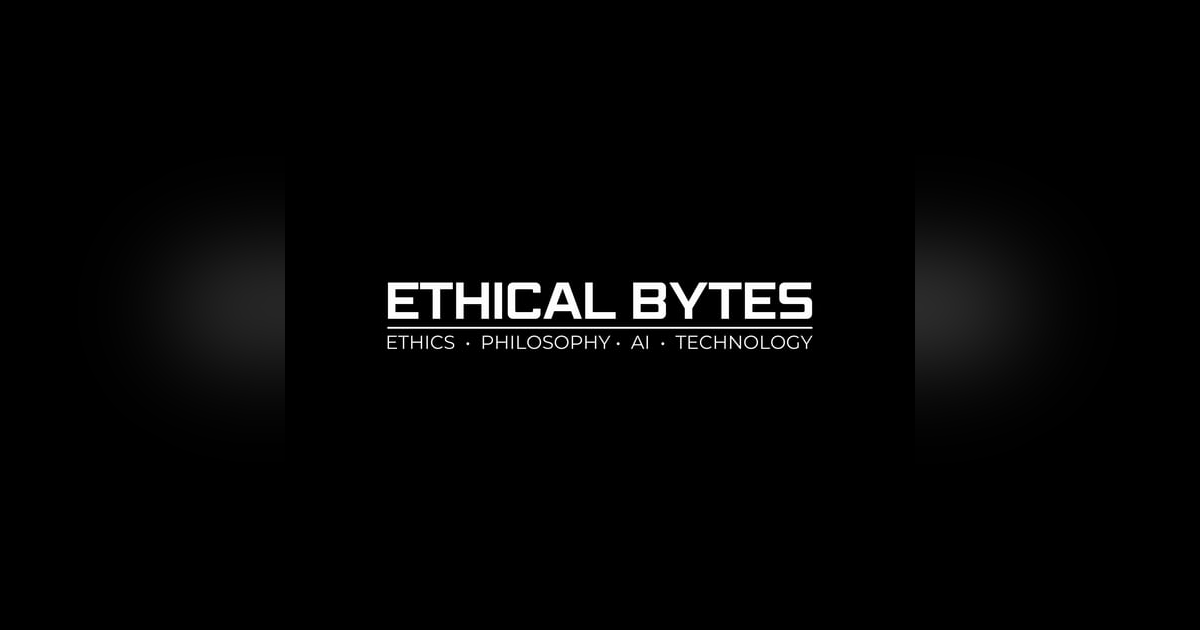
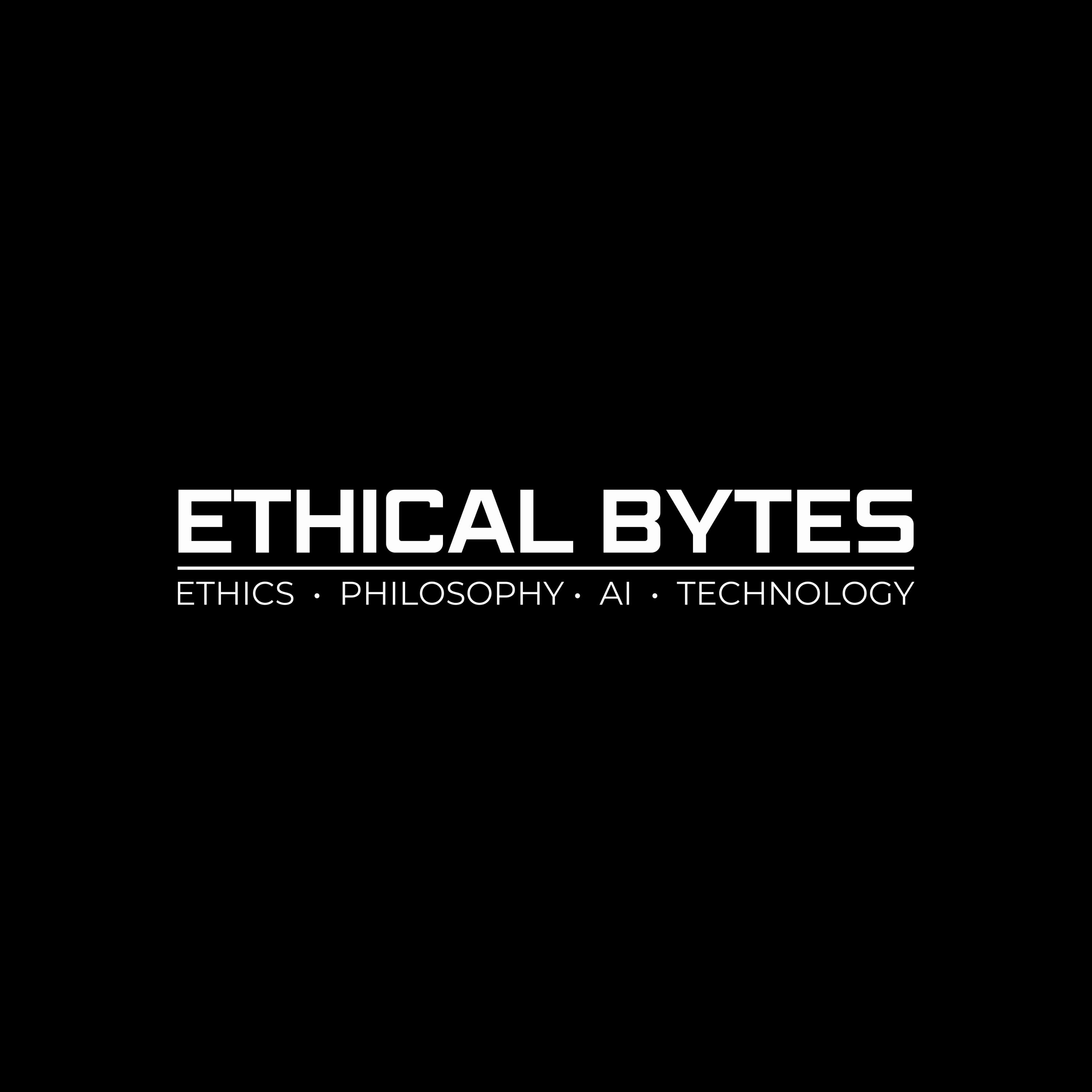
As AI continues to evolve, it’s becoming more imperative than ever to settle one of the biggest issues that coincides with–and in fact contributes to–AI development: the question of the labor behind AI. Our host Carter Considine digs into this issue.
At NeurIPS 2024, OpenAI cofounder Ilya Sutskever declared that AI has reached “peak data,” signaling the end of easily accessible datasets for pretraining models. As the industry hits data limits, attention is shifting back to supervised learning, which requires human-curated, labeled data to train AI systems.
Data labeling is a crucial part of AI development, but it’s also a deeply undervalued task. Workers in low-income countries like the Philippines, Kenya, and Venezuela are paid pennies for tasks such as annotating images, moderating text, or ranking outputs from AI models. Despite the massive valuations of companies like Scale AI, many of these workers face poor pay, delayed wages, and lack of transparency from employers.
Carter also discusses the explosive demand for labeled data, driven by techniques like Reinforcement Learning from Human Feedback (RLHF), which fine-tunes generative AI models like ChatGPT. While these fine-tuning techniques are crucial for improving AI’s accuracy, they rely heavily on human labor, and often under exploitative conditions.
It's worth repeating: We’re going to have to reckon with the disconnect between the immense profits generated by AI companies, and the meager earnings of those who do the essential labeling work.
Synthetic data is often proposed as a solution to the data scarcity problem, but it’s not a perfect fix. Research shows that synthetic data can’t fully replace human-labeled datasets, especially when it comes to handling edge cases.
It’s time to propose ethical reforms in AI development. If we want this technology to continue to evolve at a sustainable pace, we must do what it takes to ensure fair pay, better working conditions, and greater transparency for the workers who make it all possible.
Key Topics:
- “AI Has Reached Peak Data” (00:00)
- The Importance of Data for Supervised Learning (02:38)
- Digital Sweatshops (04:53)
- GenAI and the Demand for Curated Data (08:18)
- Ethical AI and the Path Forward (10:14)
- The Illusion of Synthetic Data (11:14)
- Wrap-Up: Human Labor in AI Success (12:06)
More info, transcripts, and references can be found at ethical.fm
At NeurIPS 2024, OpenAI cofounder Ilya Sutskever declared that AI has reached “peak data,” signaling an impending end to pretraining as we know it. “We’ve achieved peak data, and there’ll be no more,” he said, comparing the scarcity of internet-sourced training data to fossil fuels. Pretraining refers to the initial phase of AI model development; in the case of large language models (LLMs), like GPT, when models infer patterns from vast amounts of text sourced from the internet, books, and other media. Pretraining enables models to generate human-like responses and perform a multitude of tasks out of the box.
This declaration arrives just as LLMs and generative AI systems have started to dominate the technological and business landscape. Generative AI pretraining is largely powered by unsupervised learning—a process where AI derives patterns from these vast, unlabeled datasets. As the limits of easily accessible data are reached, however, the spotlight shifts back to supervised learning and the human labor that drives it.
Unlike unsupervised methods, supervised learning requires data that has been meticulously and manually curated by humans. Labeled data was instrumental in the previous AI boom, powering advancements in image recognition, language translation, and natural language processing. The reliance on manual data annotation has led to the rise of “digital sweatshops,” where workers in low-wage regions are paid pennies per piece of data to complete the labeling tasks required to train AI systems.
While unsupervised learning holds most of our attention in the genAI wave, supervised learning will be receiving more looks as the industry faces data scarcity, increasing our reliance on fine-tuning techniques like reinforcement learning from human feedback (RLHF) to align models with our desired behavior.
The Importance of Data for Supervised Learning
Labeled data is essential for supervised learning because it acts as “ground truth” for algorithms when detecting patterns. As machine learning pioneer Andrew Ng stated much of the intelligence and "magic" of AI models is not in the math or the architecture, but comes from the data they are trained on. While engineers can adjust model weights and parameters, the data itself has the most significant influence on how an AI behaves. However, training data isn’t just any data—it must be “good data.”
The Challenge of Creating “Good Data”
Good data labeling involves providing accurate and meaningful labels to data points and clearly describing the context so machine learning models can effectively learn and make precise inferences. Consistency is key; labels must be unambiguous and standardized across the dataset. The labeled data must be free from errors since inaccuracies compromise a model’s ability to generalize effectively. Choosing what to label is important too: labels must directly relate to the data, capturing its most important features with enough variation to represent all aspects of the task.
For these reasons, running an efficient labeling operation is challenging. Managers must create a clear method for instructing labelers to maintain quality without compromising efficiency and speed. Labeling rules and exceptions must be articulated clearly to the labelers. The work itself is tedious, detailed, and repetitive, often requiring humans to run through tons of images and text, sometimes related to profane or toxic content. The reliance on manual labor to curate data to train machine learning algorithms is more important than companies show and the burden of this crucial yet undervalued work often falls on workers in digital sweatshops.
Digital Sweatshops
The term “digital sweatshops” captures the harsh reality faced by workers who perform the task of annotating data for AI systems. These workers are concentrated primarily in low-income countries like the Philippines, Kenya, and Venezuela, where they form a hidden yet essential part of the AI pipeline. Their job involves identifying objects in images, moderating text, or ranking outputs from AI models, often for minimal pay under grueling conditions.
Workers typically earn far below the minimum wage for their efforts. Despite Scale AI’s $13.8 billion valuation in 2024, workers in the Philippines report earning as little as 30 cents for hours of work. Paul, a 25-year-old data labeler, reflected on his initial excitement about working with AI: “At first, I thought it was amazing—I was contributing to something cutting-edge,” he said. “But over time, I realized how little we’re paid for the value we create. It’s embarrassing to tell anyone how much money I make”. His story is not unique; countless labelers share this disillusionment as their expectations of meaningful work are overshadowed by the harsh realities of underpayment and exploitation.
While workers express frustration and disappointment, Scale AI’s CEO, Alexandr Wang, has publicly defended the pay disparity. He has argued that engineers deserve to be paid more because they perform the more technically complex aspects of AI development, “Engineers handle the models, the systems, and the deployment.” Wang implies that the contribution of an engineer outweighs that of data labelers. However, this perspective overlooks a crucial fact: the quality of the data, not the sophistication of the model, ultimately determines how well an AI system performs. In practice, skilled and consistent labelers are far more valuable to AI development than the engineers who design the models. Without high-quality data, even the most sophisticated AI system will fail to meet its potential.
Payment delays and lack of transparency exacerbate these issues. Jackie, a 26-year-old worker, described spending three days on a project he believed would earn $50, only to receive $12. “We’re not just numbers on a spreadsheet,” he said. “We’re people trying to make ends meet, but the system doesn’t see us that way”. Similarly, Benz, a 36-year-old father of two, was abruptly deactivated from the platform after accumulating $150 in earnings. “It’s like they can just erase you. No questions, no accountability”.
These cases highlight systemic issues within these platforms, where workers are underpaid, underappreciated, and left without recourse when payments are delayed or canceled. Paul reflected on the disparity: “We’re helping build AI that’s worth billions, but we can barely afford our daily needs.”
GenAI and the Demand for Curated Data
The explosive growth of generative AI has intensified the demand for high-quality, curated data for various techniques, especially supervised fine-tuning. One example is Reinforcement Learning from Human Feedback (RLHF). RLHF ensures that AI outputs are safe, accurate, and contextually appropriate, particularly for language models like ChatGPT. This method is labor-intensive but effective; the technique is crucial in making chatGPT both useful and trustworthy.
Other fine-tuning methods, such as quantized LoRA (qLoRA), allow for efficient and scalable training by adapting large models to new tasks or domains with significantly fewer computational resources. qLoRA adapters allow engineers to fine-tune base models without extensive retraining. Fine-tuning allows generative AI to widen its range of applicability by allowing models to be customized according to specific industries, languages, or ethical considerations.
As the number of generative AI use cases proliferates, the need for labeled data will continue to soar. Scale AI reported a jump in annualized revenue to $750 million, up from $250 million in early 2022, mainly due to skyrocketing demand from AI companies for RLHF. While RLHF, qLoRA, and other supervised learning techniques enable remarkable advancements, they underscore an uncomfortable reality: the technological achievements of generative AI are built on the labor of a largely invisible workforce and risks perpetuating cycles of exploitation.
Ethical AI and the Path Forward
The conditions in digital sweatshops raise urgent questions about the sustainability of the AI boom. The lack of regulation in these labor markets allows platforms to operate in a vacuum, setting terms that prioritize profits over worker welfare. As Cheryll Soriano, a professor at De La Salle University in Manila, observed, “What it comes down to is a total absence of standards. These platforms operate in a regulatory vacuum, exploiting workers with impunity”.
The ethical implications extend beyond labor rights. By failing to properly stymie these issues, the AI industry may risk entrenching inequities and undermining its own progress. Scale AI and in-house labeling operations must take steps to ensure fair pay, transparency, and accountability for the workers who form the backbone of their operations.
The Illusion of Synthetic Data
As data scarcity becomes a pressing challenge for AI development, synthetic data—artificially generated datasets—has emerged as a potential solution. While useful for augmenting training datasets, synthetic data cannot fully replace human-labeled data. Studies reveal that training models on synthetic outputs leads to “model collapse,” where AI systems lose their ability to handle nuanced, edge-case scenarios. Edge cases are vital for creating high-quality training datasets, meaning synthetic data only has the potential to serve as a complement rather than a replacement for human-labeled datasets. Generative AI will still require manual labor for AI training.
Conclusion
As AI continues to reshape industries and societies, it is crucial to recognize and address the ethical costs of its development. The rise, fall, and resurgence of digital sweatshops highlight the indispensable and undervalued role of human labor in AI’s success. Charisse summarized the frustration shared by many workers: “The budget for all this, I know it’s big. None of that is trickling down to us.” By improving working conditions and ensuring ethical practices, the industry can build a better and more sustainable future for AI and all the people powering it.