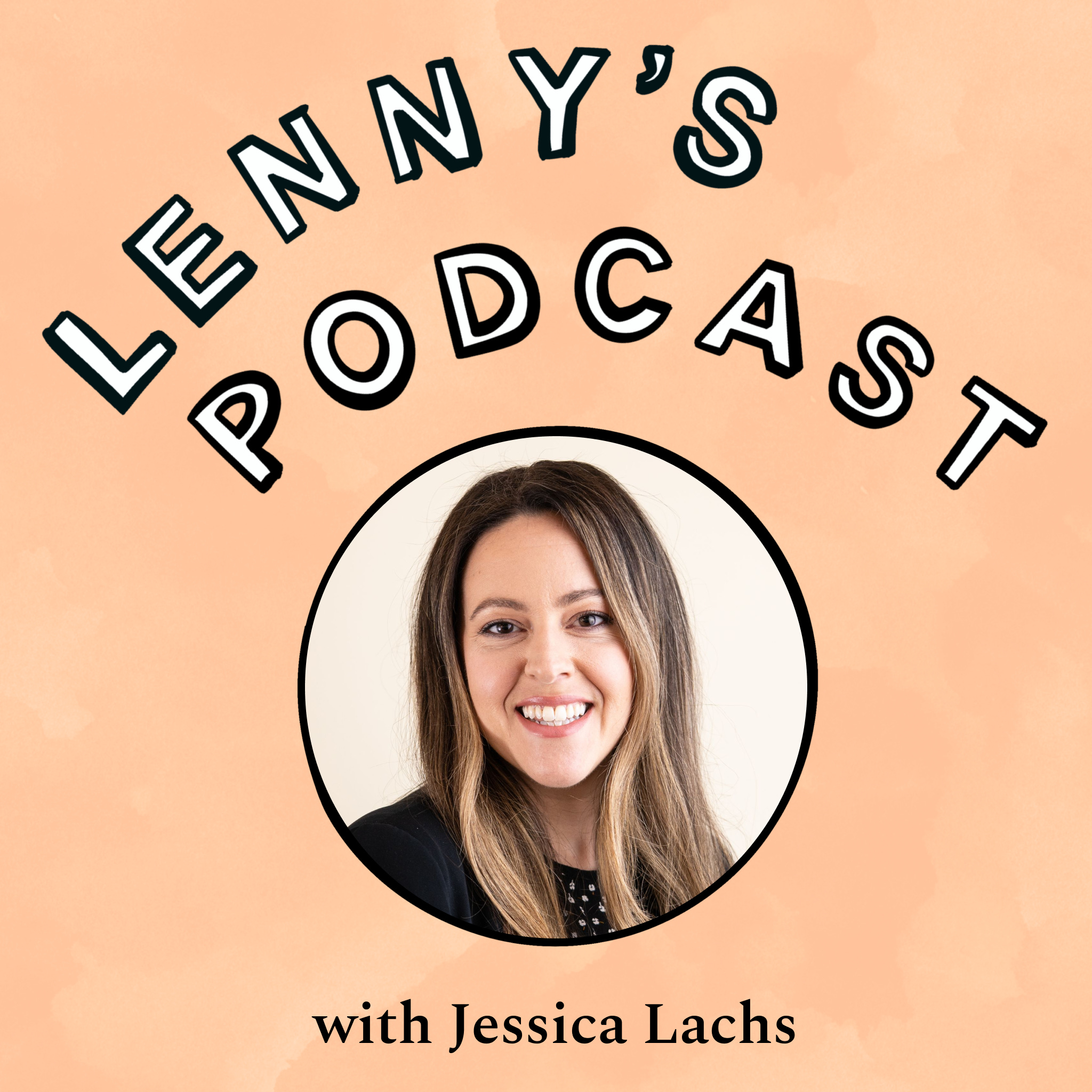
Jessica Lachs is the global head of analytics and data science at DoorDash, where she’s built one of the largest and most respected data organizations in tech. In her more than 10 years at DoorDash, she has served as the first general manager, responsible for launching new markets; the head of business ops and analytics; and the VP of analytics and data science. Previously, Jessica founded GiftSimple, a social gifting startup, and started her career at Lehman Brothers as an investment banking analyst. In our conversation, she shares:
• How to structure and scale a high-impact analytics organization
• Centralized vs. decentralized data teams
• How to pick the right metric and aligning incentives
• Advice for data people on how and when to push back
• Lessons learned from building a global data team
• How to foster a culture of extreme ownership
• The role of AI in improving analytics team productivity
• Advice for aspiring data leaders without formal training
—
Brought to you by:
• Webflow—The web experience platform
• Anvil—The fastest way to build software for documents
• Attio—The powerful, flexible CRM for fast-growing startups
—
Where to find Jessica Lachs:
• LinkedIn: https://www.linkedin.com/in/jessica-lachs/
—
Where to find Lenny:
• Newsletter: https://www.lennysnewsletter.com
• X: https://twitter.com/lennysan
• LinkedIn: https://www.linkedin.com/in/lennyrachitsky/
—
In this episode, we cover:
(00:00) Jessica’s background
(04:59) Centralized vs. embedded analytics teams
(10:52) The benefits of a centralized analytics team
(15:10) Balancing proactive and reactive work
(20:45) Advice on how to push back effectively
(24:20) Hiring for curiosity and problem solving
(28:57) Coming from a non-traditional background
(34:40) The early days and culture at DoorDash
(40:39) Encouraging cross-functional roles
(44:39) Defining effective metrics
(46:30) Simplifying metrics for better outcomes
(55:28) Focusing on edge cases and fail states
(01:00:12) Managing a global data organization
(01:02:31) Leveraging AI for productivity
(01:05:25) Building diverse and skilled data teams
(01:08:40) Lightning round
—
Referenced:
• How Netflix builds a culture of excellence | Elizabeth Stone (CTO): https://www.lennysnewsletter.com/p/how-netflix-builds-a-culture-of-excellence
• Riley Newman on LinkedIn: https://www.linkedin.com/in/rileynewman/
• Tony Xu on LinkedIn: https://www.linkedin.com/in/xutony/
• Imposter Syndrome: Why You May Feel Like a Fraud: https://www.verywellmind.com/imposter-syndrome-and-social-anxiety-disorder-4156469
• Stanley Tang on LinkedIn: https://www.linkedin.com/in/stanleytang/
• Andy Fang on LinkedIn: https://www.linkedin.com/in/fangsterr/
• Evan Moore on LinkedIn: https://www.linkedin.com/in/evanmoore/
• How WeDash became the flagship employee program for DoorDash: https://careers.doordash.com/blog/wedash-doordash-employee-program-how-does-it-work
• Leading with empathy | Keith Yandell (DoorDash, Uber): https://www.lennysnewsletter.com/p/leading-with-empathy-keith-yandell
• The Rose Code: https://www.amazon.com/Rose-Code-Novel-Kate-Quinn/dp/006305941X
• Libby app: https://libbyapp.com/
• The West Wing on Prime: https://www.amazon.com/West-Wing-Complete-First-Season/dp/B000KZPG04
• Alias on Prime: https://www.amazon.com/Alias-Season-1/dp/B00748O13S
• Joseon sunscreens: https://beautyofjoseon.com/
• Innisfree sunscreens: https://us.innisfree.com/
• John Steinbeck quote: https://www.brainyquote.com/quotes/john_steinbeck_103825
• Vanessa Roberts on LinkedIn: https://www.linkedin.com/in/vanessa-roberts-b8a509a/
• Tia Sherringham on LinkedIn: https://www.linkedin.com/in/tiasherringham/
• Elizabeth Jarvis-Shean on LinkedIn: https://www.linkedin.com/in/elizabeth-jarvis-shean-141a7966/
• Regina (Gina) Tarone on LinkedIn: https://www.linkedin.com/in/regina-tarone-a565a2/overlay/about-this-profile/
• My Journey (Part 1): I have a job that I would never be hired for: https://www.linkedin.com/posts/jessica-lachs_anniversary-datascience-finance-activity-7216912300056727553-mEv6/?utm_source=share&utm_medium=member_desktop
• Starting an Analytics Org From Scratch — Lessons From a Decade at DoorDash: https://review.firstround.com/starting-an-analytics-org-from-scratch-lessons-from-a-decade-at-doordash/
—
Production and marketing by https://penname.co/. For inquiries about sponsoring the podcast, email podcast@lennyrachitsky.com.
—
Lenny may be an investor in the companies discussed.
Get full access to Lenny's Newsletter at www.lennysnewsletter.com/subscribe
Lenny Rachitsky (00:00:00):
So you've built one of the largest and most respected data teams in all of tech.
Jessica Lachs (00:00:05):
For me, analytics is a business impact driving function and not purely a service function, not just answering the why, but answering the, "What do we do now that we know this?"
Lenny Rachitsky (00:00:15):
One of your colleagues told me that you are incredibly good at defining metrics.
Jessica Lachs (00:00:19):
Retention is a terrible thing to goal on. It's almost impossible to drive in a meaningful way in a short term. Ultimately, you want to find a short-term metric you can measure that drives a long-term output.
Lenny Rachitsky (00:00:32):
You mentioned the early team. I felt extreme ownership.
Jessica Lachs (00:00:34):
Yes, you are a data scientist, but your goal is to figure out what's happening. And if that means that you're going to pick up the phone and call customers, then that is what you're going to do to roll up your sleeves.
Lenny Rachitsky (00:00:48):
Today my guest is Jessica Lachs. Jessica is Vice President of Analytics and Data Science at DoorDash, which has built one of the biggest and most impactful data teams in tech. She's been at DoorDash for over 10 years and was the first GM at DoorDash responsible for launching new markets. Previously, Jessica founded GiftSimple, a social gifting startup and began her career in investment banking at Lehman Brothers.
(00:01:11):
In our conversation, we go deep on how to build and scale your data org, including why a centralized org model is so effective. What to look for when hiring data people, how to pick the right metrics for teams to align incentives and drive the right sorts of outcomes. Examples of how the data team at DoorDash has helped the business make better decisions, a bunch of great stories about the early days of DoorDash and a ton more. If you enjoy this podcast, don't forget to subscribe and follow it in your favorite podcasting app or YouTube. It's the best way to avoid missing feature episodes and helps the podcast tremendously.
(00:01:44):
With that, I bring you Jessica Lachs. Jessica, thank you so much for being here and welcome to the podcast.
Jessica Lachs (00:01:55):
Thank you so much for having me. I'm very excited to be here.
Lenny Rachitsky (00:01:58):
So you've built one of the largest and most respected data teams in all of tech. I've heard from a number of people that look to you for advice when they're trying to build and scale their data teams. And then DoorDash in particular is an incredibly complex business. There's three or maybe even four sites to the marketplace. There's this operational element. From the outside, it just feels extremely complicated and wild. I imagine from the inside it's even more wild. Let's talk about some of the things you've learned about building and scaling the team. You have a fairly contrarian perspective on how to structure data teams. This was referenced when we had Elizabeth Stone on the podcast too. She approaches data the same way. So I'd love to hear just your take on how to structure data teams within companies.
(00:02:42):
This episode is brought to you by Webflow. We're all friends here, so let's be real for a second. We all know that your website shouldn't be a static asset. It should be a dynamic part of your strategy that drives conversions. That's business 101. But here's a number for you. 54% of leaders say web updates take too long. That's over half of you listening right now. That's where Webflow comes in. Their visual-first platform allows you to build, launch, and optimize webpages fast. That means you can set ambitious business goals and your site can rise to the challenge. Learn how teams like Dropbox, IDEO, and Orangetheory trust Webflow to achieve their most ambitious goals today at webflow.com.
(00:03:30):
This episode is brought to you by Anvil. Their document SDK helps product teams build and launch software for documents fast. Companies like Carta and Vouch Insurance use Anvil to accelerate the development of their document workflows. Getting to market fast is a top priority for product teams, and the last thing that you or your developers want is to build document workflows from scratch. It's time-consuming, expensive, and distracts from core work. You could stitch together multiple tools and manage those integrations or you can use an all-in-one document SDK.
(00:04:04):
Most product managers will tell you, "Paperwork sucks." Anvil's document SDK helps teams get to market fast, incorporate your brand's style, and give you back time to focus on your company's core differentiated features. For your users paperwork often starts with an AI-powered web form styled and embedded in your application. From there, you can route data to your backend systems and to the correct fields in your PDFs via API. Complete the process with a white labeled e-signature. The best part about Anvil is the level of customization their SDK provides. Non-technical folks love Anvil's drag-and-drop builder and developers love their flexible APIs and easy-to-understand documentation.
(00:04:46):
Build documents software fast with Anvil, that's useanvil.com/Lenny to learn more or start a free trial. That's useanvil.com/Lenny.
Jessica Lachs (00:04:59):
There's two main things that I think are important when you're structuring a team. The first is I believe that analytics should have a seat at the table just like engineering and product and the business folks, the operators. For me, analytics is a business impact driving function and not purely a service function. I think there are analytics teams at other companies where they are answering people's questions, maybe even through Jira tickets, we're building dashboards. That was never really of interest to me. That wasn't the team that I wanted to build.
(00:05:35):
For me, it's about finding opportunities, about having a point of view on the decisions that we should make, not just answering the why but answering the so what. "So what do we do now that we know this?" And so that's definitely one thing as far as my point of view on building a data team. I think the second thing which may be a little more contrarian is I think there are people out there who think that analytics should be embedded into business units. I strongly disagree. I believe a central model, a center of excellence is superior and I'm happy to talk about why, but that's something that I feel quite strongly about. We've tried it or I shouldn't... well, we've experimented in the past with the alternative, so putting it into a business unit and it's just much more problematic and I think the value you get from a central model is far greater than some of the things that you might lose.
Lenny Rachitsky (00:06:36):
Yeah, let's definitely talk about it. And just to make sure people understand, when you say central versus embedded, is that in terms of reporting lines, in terms of their goals?
Jessica Lachs (00:06:44):
It's a great question. So mostly it's in terms of reporting lines because I think on the goal side, that is something where we have the same goals that our partner teams have, and I think that that's actually an important part of a successful central model. So when I say central model, it just means that for marketing analytics, marketing analytics is part of the broader analytics team. It does not sit and report in through marketing. Just to clarify.
Lenny Rachitsky (00:07:13):
Got it. So the reporting functions at some companies, there's the head of marketing or some partners to the head of marketing where the data, say, analyst or biz ops people or data scientists would report potentially to them and that's it. And they're not as connected to the core, to the rest of the data team, the rest of the analytics team versus-
Jessica Lachs (00:07:30):
Exactly. Yeah.
Lenny Rachitsky (00:07:31):
Yeah.
Jessica Lachs (00:07:31):
So you'd have a bunch of smaller, of course, data teams that sit embedded within the functions. And I understand why business leaders like that. You're embedded within the function, so you're a part of the team. That ownership, that camaraderie that comes with that, I think you can solve for that. But I do understand that that is a benefit. I think the other benefit of course is the business leaders control the roadmaps so they get to dictate the work. They know that they have help and resources in that area when they need them. So that certainty, that control, I totally understand the value there, but I think that those are two things that you can solve for if you know that those are the biggest issues with a central team. So for us, we have a central analytics team, but we are divided up into pods that map perfectly with how product engineering, operations marketing are structured as well.
(00:08:33):
And so our team de facto has these folks embedded with our partner teams, even though the reporting structure is up through a central org through me. And that helps the team to feel like they are one team, both in terms of the analytics team feeling like it's one team, but also to use the marketing example, the marketing folks are one team and because the analytics shares the same goals as the marketing leaders, your incentives are aligned to work on the most important things and your success is their success and vice versa. So I think that that's been really a happy medium, but still preserves all the benefits of a central org. And there are a lot of them.
Lenny Rachitsky (00:09:23):
I want to hear about them, but I think something that some people may think when you say essential org is like a silo data team that sits there and they're like a service org a little bit within the company. It's like, "Hey, I need some data help." And you try to convince that, "Hey, I need some help on this thing." And that's not what you're saying.
Jessica Lachs (00:09:41):
Oh, no. No, no, no. That job seems terrible. I don't want that job. No, to the earlier point, we have a seat at the table. We are business partners, we are thought partners with our product counterparts, with our engineering counterparts, with our ops counterparts, and we again, share the same goals and have the same initiatives that they do. And it's just our job to come at it from a data-driven place. We bring to the table insights on things that we've noticed, deep dives that we do to understand the problems that we're trying to solve better. If we need to grow, what are the most efficient ways to grow? What are the trade-offs that we have to make? Where are their pockets of opportunity? That is what I expect my team to be able to bring to that table, the proverbial table, that we want to see that. And in order to earn their spot, that's the deal. We get the seat at the table and we need to earn it by bringing opportunities that we all can go and go after.
Lenny Rachitsky (00:10:52):
Awesome. So in a sense, it is embedded. They're embedded in cross-functional teams across the org, but they report up to essential org to you essentially in the end?
Jessica Lachs (00:11:01):
Yeah.
Lenny Rachitsky (00:11:02):
Cool. What are some of the benefits of this approach?
Jessica Lachs (00:11:05):
Oh, there's so many. Okay, so the first thing is a consistent and high talent bar. I think this is something I saw when we would have some pockets of analytics folks embedded is having a consistent bar for talent in terms of what we're looking for, what are the technical skills, what are the soft skills? And being able to evaluate candidates with that same bar, using our same rubric. You just get more consistent and higher talent in my opinion. I think that's number one. Number two is actually growth opportunities. So if you're siloed, you may be the most senior data person within... I keep picking on marketing. But you might be the most senior data scientist within marketing. Where do you go from there? I think when you have the central org, you're able to see if there are growth opportunities in other areas within the company.
(00:12:04):
And so that really helps folks to stay engaged because they can look at new problems if the problems they've been working on for several years are getting maybe boring and they want something new, there's an opportunity, move from marketing over to merchant analytics. And then I think similarly, if there isn't a promotion or room to grow, if you want to be a people manager and there just isn't a people management role within your functional area, well, you've got 10 other ones to look at and maybe there is that opportunity. So I think it helps with the growth opportunities for the team, which helps to retain talent. So that's a second thing. The third thing is just consistency of methodologies and metrics. So you don't have sales that was as defined by one team and sales as defined by another team. You just have sales and everybody is using the same metrics, the same methodologies, and you're able to improve your methodologies with input from more people.
(00:13:10):
And rather than recreating the wheel, building the same churn prediction model on six different teams. You can instead build one and have the input of six different teams. I think that's definitely another benefit. Also helps you just scale because you start to see the same problems across teams and so you're like, "Ooh, this is an issue that we need to get ahead of. This is something we need to automate," or, "This is something that we need to improve upon," or, "a problem that is going to grow as our business, as our teams scales." So I think it helps you see around corners a little bit more.
(00:13:45):
And then just lastly, there's a team culture brand. I think that's really important, not just externally for recruiting top talent, but the team is really proud to be members of the analytics team. We have a unique culture of learning, of sharing. You have someone you can go to talk about your challenges. You have someone who can peer review your work. I think just having that team culture that we have is really important. And it's a lot harder to get when you have the individual silos, particularly in an earlier stage when it's a smaller team, you just don't have as many people around. Everybody wants to have friends at work and we're creating an environment where they can find like-minded data nerds.
Lenny Rachitsky (00:14:33):
It makes me think about Airbnb's first data team. I don't know if you know Riley Newman well, but he built Airbnb's first data team and it was actually an analytics team. They called themselves the 'A-Team' on the point of culture, and that always felt a lot of fun and they loved being part of that team.
Jessica Lachs (00:14:49):
Yeah. We have the same, but now I feel a lot less special for coming up with that name.
Lenny Rachitsky (00:14:55):
Oh, you called it A-Team also?
Jessica Lachs (00:14:56):
Yeah, we got the A-Team, yeah.
Lenny Rachitsky (00:14:59):
And then I think they moved away from it when there was a push. Now we're data scientists, we're not analytics or analysts. And that was like, I don't know, 10 year ago, like [inaudible 00:15:08] data science. We're data scientists.
Jessica Lachs (00:15:09):
We'll always be the A-Team.
Lenny Rachitsky (00:15:11):
There's so many threads I want to follow here, one that's a tangent, but something that I think a lot of people struggle with is you talked about how you want your data team, your analytics team to be proactive, to find opportunities, to give you ideas, to help you figure out what to build, not just answer questions. At the same time, there are many questions that teams need to get answered. Do you have any advice for just how to set up a team where they both find time to explore, dig, show opportunities and come up with big ideas and also, "Hey, we just need to figure out the funnel conversion on this thing," or "Hey, what do you think? What's happening in China right now?" Thoughts there?
Jessica Lachs (00:15:47):
Yeah, such a good question. I think it's something that never gets easier. You have to be very intentional to carve out time for exploratory work for deep dives because as you mentioned, there are always more questions and more work to be done than hours in the day. And so I think being intentional about it and setting goals for your team around finding these insights through self-directed work is an important mechanism for holding ourselves accountable to that goal because it tends to be the first thing that goes when you get a lot of inbounds, you're like, "All right, well, let's deep dive on something that I don't know if it's really something. It could be high ROI, it could be low ROI, I don't know." So the expected value is lower than this known thing that I can deliver and make someone happy.
(00:16:42):
So I think to prevent that time from just slipping away, you really have to be intentional. We would do hackathons for our team to carve out days to just go and look into these really interesting things and find opportunities. And I think we have the support of our business partners because so many great insights have come from these deep dives and it really has been some of the work that drives future roadmaps. So they're always really great at allowing us to have this time and actually encourage us often to have this time for some self-directed work, to go find the next big opportunity.
Lenny Rachitsky (00:17:23):
If there's no answer that comes to mind, that's totally cool. But is there an example of one of these insights that someone on the data team came up with that led to something big for DoorDash that you're able to share?
Jessica Lachs (00:17:35):
So one interesting example was from a hackathon we did a couple of years ago where we were looking at referral as a channel for consumer acquisition. And when you compare that channel to others, it was below average in terms of the engagement you'd see from consumers who came through that channel and the payback period. And rather than just lowering spend on referrals and moving right along, we really wanted to understand what was happening. And so during the hackathon, we did a deep dive into referral. We actually tried referring each other. We tried committing referral fraud, creating new accounts to get around rules. And we uncovered a lot of fraudulent behavior through this deep dive. We ordered so many cupcakes to the office. I remember using referral credits because you had to place an order to be able to get the referral bonus. So we would create the account, place the orders, and we just kept ordering cupcakes.
(00:18:39):
And what we noticed was that referral as a channel was a bit misleading when you would look at the average in terms of payback and that it was really a bimodal distribution and you had one group of really great consumers who were referring other really great consumers, and the payback on those consumers was really strong. In fact, if that's all you saw, you would spend a lot more on that channel.
(00:19:08):
And then what was happening was you had this other group of consumers that were not as good people who were posting referral codes online and getting people who were just in it to get free discounts and credits. And we had at that point in time, pretty lax fraud rules. And we didn't have caps on these things. All of which came about from this deep dive where we found that this group of consumers was really a drag on the efficiency of this marketing channel. And so I think that's an example of a few things that we like to do at DoorDash. One being these deep dives and taking the time to really understand the problem and then ultimately make a bunch of recommendations for what we should do, including better fraud checks, caps on referrals, et cetera, et cetera. But also how the average can be incredibly misleading. And so looking at distributions and trying to break down what you're seeing to find ways that you can optimize in ways that you can gain in efficiencies.
Lenny Rachitsky (00:20:21):
That's an awesome story, great memory to come up with that one. So this is a really good example of a way to carve out time for the data team to think long-term, think look for opportunities, find big ideas. So the hackathon is one idea. Imagine many data people are struggling often to push back on asks that are just like, "h, we need to know. We just need this one thing. Here's a question, just answer this one question part." Do you have any advice to data to get better at pushing back? Sounds like a bit of cultural like, "We have time, we need to work on these bigger things." But just any advice for data leaders or data ICs to find time for these sorts of things?
Jessica Lachs (00:20:59):
Yeah, saying no to someone is never fun. I think as a self-proclaimed people-pleaser, you don't want to say no, especially when it's something you can do and you know that you can very easily with maybe an hour's work, make someone happy. I think it's really important to establish a culture and for leadership to really establish the rules of working and that operating model so that some of the junior folks aren't forced to always have to say no. And I think one of the ways we do that is through our goaling. So because our goals are the same as our business partners, we're able to pretty easily say, "Hey, we've got a limited amount of time. These are our goals. What are the most important things that we are going to work on this week or this month in order for both of us to hit our goals?"
(00:21:49):
And so when something comes up to be able to say, "Hey, this data poll that you want me to do, is this more important than these other three things that I was going to be working on? Yes or no?" And I think sometimes people don't necessarily realize the trade-offs, and when you make them apparent and you put them front and center, they realize that, "Oh, actually, you know what? That asset's not important. That can wait." So I think that that's definitely something I would recommend, which is always share the trade-offs. Don't suffer in silence with, "How am I going to do all four of these things?" Bring it up and say, "Hey, this is what I was planning to do. If you want me to do this extra new thing, then one of these other things is going to have to drop.
(00:22:36):
I personally don't think that your ask is more important than these three things, but maybe there's new information, maybe there's context I don't have, so let's talk about it." Rather than just being like, "No, I won't do that." That's not a great approach either. I think having the conversation and constantly reevaluating your prioritization to make sure you're working on the most important things or your team is working on the most important things is really good hygiene to have with your business partner. So some teams do that through a weekly standup like, "Here's what we're going to do this week. Do we like this prioritization? Do we not?" Some folks do it less formally than that. I think you got to figure out what works for you. But to the earlier point, it's a conversation with your engineering partner, your product partner, your ops partner, you're all on the same team, you're all trying to achieve the same goals and you're all incentivized to have your analytics team working on the most impactful things.
Lenny Rachitsky (00:23:32):
This advice is great for any role basically. And if I were to summarize it to a couple words, it's just prioritize and communicate what your priorities are and then align on the trade-offs of shifting your priorities.
Jessica Lachs (00:23:45):
Every once in a while you just throw one over and say, "You know what? This is quick. I'll do it." At least I do. I think sometimes just knock it out, build some goodwill. I think that that's also important. But usually it's not something you can do in five minutes and in that case it's that ruthless prioritization for sure.
Lenny Rachitsky (00:24:05):
And then there's also the side that you talked about of just show that you can provide value doing these things that are longer term, like prove your worth. "Hey, look at all these opportunities I found for our team over time, I should keep spending time on these other areas," versus the on fire stuff.
Jessica Lachs (00:24:18):
Exactly.
Lenny Rachitsky (00:24:19):
When you're hiring people for your team, I'm curious what you look for and you think is incredibly important that maybe other people aren't prioritizing as much. What do you focus on when you're hiring?
Jessica Lachs (00:24:31):
Yeah. So everybody needs to have a certain set of technical skills. I think that's a non-starter. We have a technical bar, we do a technical screen. So I think that's table stakes. There's some really unique characteristics that I've noticed when I look at some of the top talent that I've had on the team or have on the team. I think the first thing is just curiosity. You can't teach curiosity, or at least I haven't found a way to do it. If somebody else knows how, please let me know. Somebody who is just self-motivated to pull on the threads when they find them. So they don't just answer a question. They're like, "Hmm, this thing seems a little odd. I'm going to dig in and look. Even though I could say I'm done, I answered the question, I did the thing I was going to do." The person that has that curiosity, something seems off, something doesn't really make sense and goes and proactively looks into what that is. That is just so valuable. So I really look for that curiosity and that self-motivation to do it without being told.
Lenny Rachitsky (00:25:39):
How do you test for that? How do you do that in an interview and get a sense of if they're good at that?
Jessica Lachs (00:25:43):
One way you can do it through the questions you ask is have something that is not quite right within the case that you're presenting and see if people notice first and foremost. And even if they don't, if you point it out like, "Where do they go with that?" I think that that's something that you can test for. I think you can also ask for examples that for these folks typically will highlight this, they'll talk about, "I noticed this thing, and so we decided to investigate." So I think that there are ways that you can get that signal through the interview process, but it's really hard. I think testing for hard skills is a lot easier than testing for soft skills. And I think in some of the questions we ask, we'll ask a question with the idea that we're assessing something separate than what the question is necessarily asking. And I think that this is one example of where that really works.
Lenny Rachitsky (00:26:47):
You said that you give them a case. What does that look like? What is the actual approach to how you do this interview?
Jessica Lachs (00:26:52):
Our interview process has in the early stages a coding exercise. So we do our technical screen and a shortened version of a business case. So real world problem solving. Typically, it's something actually from DoorDash history, like a real problem that we had to see how people can problem solve on the fly. I think that that's an important skill to be able to have, which is, how do you take a problem, break it down, talk through it. A little bit like some of those consulting cases that you hear about, but something that's really rooted in real problems. And I think you can learn a lot from those types of cases where, yes, you get to see how people handle ambiguity and structured problem solving, but ultimately most people get something wrong. They make an assumption that's wrong because well, I would hope that the interviewer knows the business better than the interviewee.
(00:27:56):
And seeing how people react to being told they're wrong is a really important signal in my opinion. Seeing how people respond, how they're able to take new information and pivot, how they're able to make a decision. So that's another thing that I like to see in cases where, hey, you may not know the real right decision. You might say, "Hey, I could see it going one way, I could see it going the other way." But I always push people to say, "If you had to make a call right now, what would it be?" So are people able to have a point of view without full information because that's life. Sometimes you have to just pick a direction and make a decision even though you don't have perfect information. So I like to see some of these softer skills and how they manifest throughout a case interview, even if it's not specifically what I'm asking with the literal problem we're solving in the case.
Lenny Rachitsky (00:28:57):
Along these lines, but in a different direction. You don't actually have a deep data science data background before you got into this stuff. I know you had some art background, you had an art portfolio back in school, and I think a lot of people wouldn't imagine that for someone being head of analytics for a company like DoorDash. I don't exactly know the question, but I guess is there anything there that you think would be interesting for people to know or hear?
Jessica Lachs (00:29:24):
Yeah, it's funny. I joke that I have a job I'd never be hired for because I don't have a traditional data science background. And I know that Elizabeth Stone on her podcast with you talked a lot about her non-traditional background for a CTO. So hey, maybe there's something to it. But I became a data scientist out of necessity. I completely self-taught in terms of SQL and Python and I did it because there was a need at DoorDash for someone to help figure out what the right goals were, how we set those goals, how we were performing different markets early in the DoorDash story, so 10 years ago at this point. And I think I just gravitated towards that type of work and Tony recognized that superpower in me even though I don't have that formal training. So yeah, I'm a bit of an artist for fun, but I guess a data scientist in practice or for career.
(00:30:31):
But I think that that non-traditional background has been a great thing because I'm able to hire people who have the technical skills that I don't have, the folks with PhDs in statistics and the data scientists, machine learning and otherwise. I am able to hire those folks and yet keep them really focused on driving business impact because my background was on the finance side, and so I've always been a pragmatist. And for me, the purpose of our team is to drive business impact. And so the mix between the technical skills of the smarter people that I've hired, the smarter than myself, and my grounding in driving business impact has been a really great partnership.
Lenny Rachitsky (00:31:21):
That's quite an inspiring story for someone that is just starting out and doesn't necessarily have a lot of experience in data, but also just generally. I think this is a really cool example. You could be successful in a field that you don't have a ton of background in. I'm curious what you think it was in you that allowed you to succeed in this and get to where you are today. What do you think you did right or what is some habits or ways of thinking that you think helped you achieve that?
Jessica Lachs (00:31:52):
First off, I have imposter syndrome like everybody else. So it's not like I have this crazy sense of confidence of like, "Oh, I can do anything." I definitely have the same doubts that others have. I think part of it was probably not even realizing what I was doing. When you're at a startup and things are moving quickly and you see a problem, and I've always liked solving problems, so I was like, "All right, how do I solve this problem?" It was like, "Oh, well, I need access to the data. I don't have access to the data. All right, I'll ask an engineer to get me the data. Well, this isn't going to scale. I can't always bother an engineer, so how do I figure out how to get the data myself? Well, let's learn Python." So I think it happened organically and I don't think I realized at the time what I was even doing.
(00:32:41):
And then I think if you think about things from first principles about what you need right now in front of you to unblock yourself or solve a problem, and you just focus on that instead of thinking about a global org that you're trying to build. I think that that helps. So for me, it was always about solving the problem in front of me the best way I could. And if that meant I needed to hire an engineer to report into me through the finance org, then that was what we were going to do and nobody was going to tell me I couldn't do it. So I think it's a belief in yourself, and ultimately it's just my desire to solve problems and figure out what has to get done is, I think, ultimately how it came about.
Lenny Rachitsky (00:33:30):
I love that so much. There's so many elements there that I think a lot of people can learn from. I feel like there's also this underlying current of you're just motivated for this to work. You wanted DoorDash to succeed, and you're just like, "I will do what I need to do to make this happen. I need to solve these problems. I'm not going to overthink. Do I have the skills necessarily to do these things [inaudible 00:33:48]?"
Jessica Lachs (00:33:48):
Yeah, I think I'm competitive. I think that's a trait that you find in a lot of early DoorDash folks and current DoorDash folks, to be honest, just wanting to win and being willing to do whatever you need to win. So roll up your sleeves, do something that's not your job. I think back to early days of taking out the garbage on Saturday nights because it needed to get done. I think that that was something that is ingrained in our culture from Tony Xu, from our founder and CEO, and I think that really resonated with me, and I feel like I've always operated that way as well. And I think that that helped me in my career to be able to do what I've done without really thinking about it too much.
Lenny Rachitsky (00:34:40):
Are there any other memories or stories of the early days of DoorDash that would be fun to share? Something that sticks with you of like, "Wow, I can't believe that's what it was like?"
Jessica Lachs (00:34:49):
Oh man, there's so many, including so many mistakes that we've made. But I think something that really stands out to me is before I moved to the analytics area, I was actually a GM. I was the first GM at DoorDash and I was in Boston in 2014 launching the city of Boston when nobody knew who we were. And we would wake up early in the morning, 5 A.M. and we would go out, it was the winter of 2014. We'd go out and we'd hand out promo codes consumers outside of the [inaudible 00:35:30] in Boston, and these promo cards would be attached to kind bars so people would take them. And the whole team, it was a small team, there were four of us, but the whole team would go out in the morning to do this. And I think back to our sales guy, shout out to Joey G. So Joe Graccio is our sales guy in Boston-
Lenny Rachitsky (00:35:47):
[inaudible 00:35:47] Joey G.
Jessica Lachs (00:35:51):
And he was gold on signing merchants on the platform.
(00:35:55):
That was how he was gold. His compensation was tied to that. And yet in the morning when we would go out, he was with us handing out promo codes because he was part of the team because he wanted to win. We wanted to grow the business. And I think that that is just a great example of the culture that Tony and the early employees and Stanley and Andy, other co-founders really instilled in all of us early in those days. So I think that that ownership, that extreme ownership of the outcome is definitely one of the things.
(00:36:32):
I think the other is just being very customer first. And I say customer, I mean consumers, dashers and merchants as all being our customers. And the first time I ever went to the office headquarters in Palo Alto, which at the time was in an animal hospital. The first time I went there, there was a huge site outage and the whole company, it was like 20 people at the time, the whole company jumped online to do customer support, to answer the phones, to make sure that folks were getting refunds for orders that weren't going through, make sure the orders that were out there were getting delivered, just dropped everything and hopped on to do support.
(00:37:15):
And I was brand new, didn't really know how to use the tools, and so it was like, "How can I be useful?" And so back in those days, we used to order dinner to the office using DoorDash. And so in order to preserve about three dashers who would've had to deliver food to us, I was like, "I'm going to go out, go out dashing, go get everyone pizza so that we could feed the masses doing credits and refunds and do what we had to to make sure that we were serving our customers well." And I think that night was one of the largest refunds as a percent of our bank account that we had ever given out. And I think Tony, there were two examples that he's talked about where we just gave a lot of money back to customers because it was the right thing to do because our service failed and we wanted to do right by them.
(00:38:06):
So I think that those are two stories that stick out in my mind and really highlight culturally what makes DoorDash unique and what I think has been a really important part of our success.
Lenny Rachitsky (00:38:20):
It reminds me of the story that Tony and all the early employees, and I imagine you did this just like, "We're dashers," it's like a rotation where you dash for a while. Is that part of the culture?
Jessica Lachs (00:38:32):
Yeah, so we have a program, a WeDash program, and Keith Yandell, who's our chief business officer, did your podcast last year and he talked about this. But four times a year all the employees go out and go dashing or do customer support, and it's part of our culture that I love. I actually go pair dashing, so I go together with one of my colleagues. We've done it for years now, and it's a fun thing that we do together four times a year. Actually, usually more than that. And it's important because you get to use the product, you build empathy with all the audiences. I think all of us order DoorDash a lot, so we've built empathy with consumers. But being able to go and understand what it's like to go out dashing and when you're in the restaurant going and talking with merchants and seeing the experience from their point of view, I think it's just incredibly important. And of course we find a lot of bugs like, "Hmm, this doesn't work the way it should, let me report this." So I think it's also just great for catching bugs in the product.
Lenny Rachitsky (00:39:43):
This episode is brought to you by Attio, a radically new type of CRM. There's a world where your CRM is powerful, easily configured, and deeply intuitive. Attio makes that a reality. Attio is built specifically for the next era of companies. It syncs with your data sources, easily configures to their unique structures and works for any go-to-market motion from self-serve to sales led. Attio automatically enriches your contacts, syncs your e-mail and calendar, gives you powerful reports and lets you quickly build Zapier style automations.
(00:40:17):
The next era of companies deserves more than an inflexible one-size-fits-all CRM. Join modal, replicate 11 labs and more, and scale your startup to the next level. Head to at attio.com/Lenny and you'll get 15% off your first year. That's attio.com/Lenny.
(00:40:39):
I want to come back to a thread, something you mentioned where you and a lot of the early team had felt extreme ownership over the company and that's why a lot of this stuff happened. For people, every founder, every product team, they're going to like, "Yes, we need that. Let's make sure everyone on the team feels extreme ownership." Is there anything that you think that the early team did to create that or is it hiring, just pick people that will have that feeling already, or is cultural?
Jessica Lachs (00:41:05):
I think it's both. It's definitely cultural. I think it comes from the top and I think that Tony exhibits this extreme ownership and looks for it in others. So I think that helps. But I think even today I expect of my team that same extreme ownership over the outcomes. And so I'm more interested in our team figuring out how to solve a problem than the box that someone fits in like, "I'm a data scientist and so I only do these things." Right? It's like, "No. I mean, yes, you are a data scientist, but your goal is to figure out what's happening, and if that means that you're going to pick up the phone and call customers, then that is what you're going to do." And I think that expecting that and setting that as the norm for the team, this ownership of the outcome is something that we continue to do at DoorDash and instill in everyone whether you were early or just joined last month.
Lenny Rachitsky (00:42:09):
Is there an example of that that comes to mind of someone practicing extreme ownership, like a data scientist calling someone or something along those lines?
Jessica Lachs (00:42:16):
Yeah, so I actually had a meeting yesterday morning with the team that's working on some of our affordability initiatives and we had shipped something that we expected to work, and it didn't. And instead of, "You can dig into the data," to understand the segments of consumers that you would expect it to work with and those that it wouldn't, of course we did that. But ultimately it was like, "I don't know why." And that's where qualitative research is superior to quantitative research, it's asking for the context, to actually talking to people to figure out what was the motivation, what worked, what didn't for them. And so the team, data scientists included, just sat and made phone calls. And so they were talking about what they found from those phone calls and that's going to inform future decisions. And I think rather than saying, "Well, that's what the qualitative research team is supposed to do," it's like, "No, no, no, that is what our team, anyone's team is supposed to do because that's what's needed to unblock us from this next test that we want to run because we need to know what we are testing."
(00:43:23):
So I think that it happens every day. I think I really love when I see team members go outside the traditional bounds of what a data science role might be and do some product management work, do some engineering work. I think that that's part of what keeps the job interesting. I think it's part of what makes our team special is that that is not only allowed, it's encouraged, and probably also a reason why we've had folks who've gone from my team to the product org and to the ops org and to the finance org is because they get to do and experience parts of that job and get a good sense for what that's like and then realize it's something that they love. So I think it's definitely something we encourage at DoorDash.
Lenny Rachitsky (00:44:17):
I love that. I want to move in a slightly different direction. One of your colleagues told me that you are incredibly good at defining metrics, which is so important to get right for a business, especially when it's complex at DoorDash. And I hear you're especially good at finding the right metric to drive the right incentive, especially when the business is really messy and things like that. So I'm just curious what you've learned about how to pick good metrics and align incentives well.
Jessica Lachs (00:44:45):
I've learned a lot of things about metrics, mostly from bad metrics. I actually think you learn a lot from picking the wrong metric. Ultimately, you want to find a short-term metric you can measure that drives a long-term output. So people always talk about, "Oh, we want to drive an improvement in retention." Retention is a terrible thing to goal on because it's almost impossible to drive in a meaningful way in the short term, and yet you want to be able to experiment and iterate quickly. So what are the things that drive retention? What are the inputs? So I think it's really important to find the right inputs, and then through experimentation test whether or not those short-term inputs are driving the long-term output that you're looking for. I think that's one thing. I think keeping things simple is another thing I've learned over the years, maybe it's data scientists, but they tend to love these composite metrics with a coefficient.
(00:45:44):
"We're going to wait this input at X and this input at X+2." And then you end up with a metric that nobody really understands that doesn't actually mean anything. And you're like, "I don't know if a 0.1 increase is it a lot? Is it good? Is it bad?" So they're just hard to work with.
(00:46:07):
And so I always encourage folks, just pick something simple, even if it's not perfect and your composite would be more perfect. If people understand it, if they have an intuition around it, if it's something that people can talk about across the company, it's going to be a much better metric in terms of driving real outcomes than your made up composite score that nobody understands. So I think keeping things simple is also really important. And then I think the last thing I'll say is it's important to understand how metrics across the company equate to one another.
(00:46:43):
And so we spend a lot of time quantifying things in terms of a common currency. So for example, if I were to lower price by a dollar, what would I get in terms of, we'll say, volume? Well, what if I lowered delivery times by a minute? What do I get for that in terms of volume? And so now you can make trade-offs between maybe your marketing team and your logistics team because you have this common currency that everyone can talk about. And so we've done that. We've tried to quantify all of the levers of our business, price, selection, quality in common terms, so that if we have, say, a dollar to spend, we know what we get depending on where we put it, over what timeframe. I think that that helps us make decisions more quickly because we know what our options are. We know we have our inventory of things that we can do, short-term, long-term, and what we get for it. So it definitely helps us to make decisions more quickly and hopefully better decisions.
Lenny Rachitsky (00:47:56):
These are so awesome. I definitely want to follow up on some of this. This is so good. So maybe on this last one, which we did at Airbnb also like, how does everything translate into Knight's book and booking? Every decision we make, what is the actual Knight's book impact? And so I imagine in your case, I don't know if you want to talk about these things. I imagine it's transactions, or purchases, or GMB or something like that, so I'm guessing is the final metric. I don't know. Is that something you talk about or you don't talk about that?
Jessica Lachs (00:48:26):
We measure things in terms of GOV, so Gross Order Value, and also volume.
Lenny Rachitsky (00:48:33):
Got it. [inaudible 00:48:34]. Okay, so basically every other metric that people are gold on as much as you can translate, there's a model that translates that into Gross Order Value and volume? Awesome. So when a team is saying, "Hey, we're going to change the onboarding flow and impact conversion here." I don't know. I guess what are some examples of other metrics on teams that potentially translate into GOV and volume just to make it even more real?
Jessica Lachs (00:49:01):
Yeah. So everything from the example that you started with, which is an improvement in the login flow, how many more consumers are getting onto the app and ultimately placing orders. And so you can translate that to, of course, orders and GOV. But then something as interesting as selling a Thai restaurant in Sacramento, we're able to say, "What do we think that that gets us in terms of GOV from the consumer by selling that Thai restaurant?" So it's every area of the business, it's mobilizing more dashers on the road. What does that do to our quality metrics in terms of delivery times? How does that translate? So because of that, we're able to figure out if we want to spend the dollar or spend the time, the team's time, on improving conversion or spending more money in marketing or onboarding more dashers or signing more restaurants or adding more grocery stores. So we are able to look across the whole business and figure out what is the right mix of actions to take to achieve our goals.
Lenny Rachitsky (00:50:18):
I could see as you talk about this why this is so important in a marketplace, especially a multi-sided marketplace where there's always trade-off decisions between supply investment and demand growth and dasher growth. I don't even know, my brain would explode trying to think about all these things, so I get exactly why this is so important to business. Okay. And then in terms of the simple recommendation, I think when people hear like, "Yeah, keep it simple," they're like, "Yeah, yeah, we're going to keep it simple." What are some things that point to, "This is not simple," that tell you like, "No, this is way too complicated. You should try to simplify this metric even though it's not ideal. It's not the perfect metric, but it needs to be simpler."
Jessica Lachs (00:50:56):
Yeah. So we had a score for merchant health, which we tried experimenting with, which was a combination of factors that we had found would lead to a merchant being on the platform and getting an order. So we wanted to make sure that the merchant had active hours on the platform and had images and had a full menu that was accurate and robust. A number of different inputs. And we created a composite that weighted all of these different inputs. And then we were like, "What is our merchant health score?" And you were like, "It's 0.35. It's not 35%. So what is that, that 0.35? I don't know what it is." So instead of that, we said, "What are the most important factors in order... First, let's measure how many of the new merchants are getting an order within their first, say, seven days on the platform.
(00:51:57):
And then let's look at how many of our merchants are doing these things we know are important. So these inputs. So let's goal our team on getting merchant photo coverage up. Let's goal the team on making sure that we have open hours, accurate hours." So yes, someone might say it's simpler to have a composite metric, but it was so hard to understand what it was and how to move it that it became meaningless. And ultimately moving to something that was simpler to understand, even if it meant having three metrics instead of one, it ultimately was better for the team because folks knew what they were trying to move. And so yeah, maybe we missed number four, five and six on the list of things, but you got one through three and that's 95% of it anyway. So once we get success with that 95, then let's talk about figuring out the other 5%.
Lenny Rachitsky (00:52:55):
It's so funny because this is exactly what we went through at Airbnb, we had, we call that a healthy host. I led the host quality team for a while and we came up with this healthy host metric that was six factors of a host, like the cancellation rate, the review rate, their response rate and things like that. And then we're just like, "Cool, let's move this, let make more hosts healthy." And then you end up like, "Okay, which one do we focus on?," And, "Oh, what about all these others?" And we ended up basically focusing on one at a time. And so let's just make that the goal for now and then rotate through the different biggest [inaudible 00:53:28] opportunities to move. [inaudible 00:53:30].
Jessica Lachs (00:53:30):
Exactly. I think in hindsight for the example you give, which of those six things are actually the most important? And if you're able to then quantify which one matters most, you work on that one first and you materially move that one and then you work on the next one. You want to move them all. But being able to prioritize and know what you're going to get for a 20% improvement in, say, your cancellation rate, that's where analytics I think can add a lot of value. Because yes, ultimately you'll get to all of them, but the way you do that and the time can have a meaningful impact on your growth. If you can target the most problematic things first and solve those, you get more bang for your buck and that compounds over time. And so doing the things that matter first and most quickly is a competitive advantage in my opinion.
Lenny Rachitsky (00:54:21):
The other thing we found along those same lines is rotating between different metrics is so not efficient because you get good at, "We're going to move this metric." And your team's like, "Cool, we totally understand this lever," like cancellation rate. We become really smart at cancellation rate and then three months later, you need to switch to response rate and they have to learn a whole new paradigm of how to think about it. And it's just super inefficient. So we found basically, just keep a team on the metric until there's no more opportunities and give another team one of these other metrics.
Jessica Lachs (00:54:50):
Yeah.
Lenny Rachitsky (00:54:52):
So many lessons. Okay. And the first thing you said on how to pick a good metric about this idea of short-term metrics that have long-term impact. How did you phrase that again?
Jessica Lachs (00:55:02):
Yeah, so we find proxy metrics for long-term outcomes.
Lenny Rachitsky (00:55:05):
Awesome. And it's similar to the simple metric, and it all comes down to, again, just like the metric should be something probably, you can move, you can understand, that's close enough to this ideal, perfect metric, but isn't necessarily the entire ideal. Okay, awesome. Anything else along these lines of just picking metrics, working with metrics that you've learned that would be worth [inaudible 00:55:28]?
Jessica Lachs (00:55:27):
With metrics, we are often looking at the average, and I think we talked about this a little bit earlier, but making sure that you're looking at the edge cases and your fail states is also really important. And so we often will set goals actually and create metrics around those edge cases. So like the disaster deliveries, the ones that go terribly wrong. So we have this concept of Never Delivered, which is orders that are never delivered. We're really great at naming things at DoorDash, and they're very rare. And so if you were just looking at the average effect or the average consumer experience, it would never come up. If you were just measuring quality based on average values of delivery times and lateness [inaudible 00:56:18], these wouldn't show up because they are so rare, but they're terrible. They're terrible experiences for consumers. They lead to churn.
(00:56:27):
They're incredibly expensive because you're refunding an order or repurchasing food and having to send another dasher to deliver that repurchased food. So they're very expensive, they're costly from a consumer experience standpoint. And I think if you're not looking for these fail states, they are often missed. So I think when you're picking metrics, yes, you want to improve engagement and you want to improve conversion, and there's a lot of things that are averages overall that you want to move, but it's so important to find these edge cases in these fail states and actually set concrete goals around eliminating them because it can be really powerful.
Lenny Rachitsky (00:57:11):
So the tip here is actually make that a goal like, never deliver at some team, just keep cutting that down?
Jessica Lachs (00:57:17):
Exactly. So we have part of our quality analytics team and we have product engineering and ops on it as well. Their goal is to eradicate Never Delivered. And in order to do that, you have to understand why they happen. Sometimes it's human error, sometimes it's fraud. And then figure out ways that you can prevent them, that you can fix them while it's happening and ultimately just get rid of them from the system. And you're never going to completely get rid of them, but you can make a meaningful impact to make them even more rare than a fraction of a percent.
Lenny Rachitsky (00:58:00):
Yeah. And I feel like people may be hearing this and like, "Of course, why would you not focus on terrible work experiences?" But I think in most companies, they look at the big numbers, they look at the averages as you said like, "Oh, it almost never happens. Why do we even spend any time on this?" And your point is, you should actually spend time on these really terrible experiences, even if it's a tiny portion of your business. I guess maybe share why that's important. Is it just because that has trickle-down effects on the brand?
Jessica Lachs (00:58:27):
Yeah, I think it's a couple of things. So just because something doesn't happen frequently doesn't mean that it's not important. So the Never Delivered example is a great one in that this is leading directly to churn and it's also costing a lot of money far more than its frequency would suggest. And I think the fact of the matter is is when you have things that cause churn, you're losing all of that consumer's subsequent orders, and that is not necessarily observed. You're just seeing one bad experience, you're not seeing all of the lost orders because they're lost. And so I think that sometimes this is an area where the data doesn't show you the full picture. And being able to quantify the impact on engagement, on profitability, will make it stand out as something that really, that you would maybe miss if you weren't really looking for it.
(00:59:25):
And then I think the other thing is with something like login errors, sometimes you don't see it in the data because people can't even get into the data. If you're not able to log in, you're not making any purchases, you're not ordering, and so you may not see it in the data that you're looking at. And so that's also something that I think is important for data folks to think about, which is what data don't we have? What data might we be missing? Where might there be opportunities and things that we actually need to identify and fix that we may not see? Because in this case, with login failures, they're not able to log in. They're not in the denominator, and so we're missing out on them from the data set entirely.
Lenny Rachitsky (01:00:15):
Just a couple of more questions. There's one that I skipped that I'm just going to come back to. It's completely out of nowhere, but I think it might be interesting is about a global data org. So you run a global data org, you have data scientists and analysts and biz ops people all over the world, not just the US. I'm curious just how is it different managing data people in different countries versus just the US? What's a big difference?
Jessica Lachs (01:00:37):
Everyone always asks about the differences. What I am surprised by is how similar things are, how similar people are, the data scientists themselves, but also consumers and dashers and couriers, as we call them at Volt. There's a lot more similarities than differences. I do think that when you built a business in the US and then you introduce new countries, having different currencies and different languages adds complexity that you weren't necessarily familiar with. I think similarly in EU countries versus non-EU countries in Europe, there's different regulation. So that adds a fun layer of complexity. So I do think that it adds complexity to the problem set, but ultimately so many of the problems are the same. It feels a little bit like going into a test having seen the answer key. And so for me, there are problems we've encountered at Volt through Volt analytics where I'm like, "Oh, we've had a similar problem.
(01:01:49):
I have an instinct for what the answer might be. Let's still test because there could be differences cultural or otherwise, but I feel like I know where we're going to end." And then sometimes there are problems where it's new for one reason or another, and it's exciting because you're like, "All right, let's see if things are different here." Let's see what ideas might work in a Volt country that don't work in a DoorDash country and vice versa. So I think I tend to focus more on what's the same, and then I'm pleasantly surprised when I find things that are different because that keeps you on your toes and keeps things interesting.
Lenny Rachitsky (01:02:31):
I'm going to take us to AI Corner. This is a segment we have in the podcast where I try to understand how people are using AI in their day-to-day and in their business. I'm curious if you've found some really interesting way of using AI ideally in... you can go in either one of these directions, and how you or your team work day-to-day using AI tools to make you more efficient, or integrating AI into your product, making DoorDash better.
Jessica Lachs (01:02:59):
Yeah, I think that there are opportunities in both. I think one of the things I'm really excited about is actually the former. So in helping to make the team more productive, we do something called Office Hours at DoorDash, the analytics team. And it's something that we started eight years ago, and it was a way to provide support for teams that at the time we just didn't have the bandwidth to support. So we would go, in the early days, we'd go sit in a room and we'd say, "Come on in and we'll help you with anything you need help with. We'll help teach you SQL. We'll help look at some of your work. We'll be a thought partner. You could just come learn what we're working on." Whatever it was. We would do two hours every week of Office Hours at different times to be friendly to different time zones.
(01:03:52):
And I think one of the things I'm excited about is being able to really empower some of the folks that are still coming to Office Hours for one thing or another to be able to use AI to help edit queries on their own for example, to be able to say, "Here's a query. I want to make this. Please adjust this to our grocery business so that I can see the GOV for grocery." And so working to build these tools that will help not just our team in terms of time saving, and also to be honest, folks are going to use it on our team, but really to be able to empower non-technical users to be able to do things on their own and not have to take up bandwidth for the analytics team.
Lenny Rachitsky (01:04:40):
So essentially it's a chatbot that anyone in the company can talk to you to get advice on how to write SQL queries, query data and things like that?
Jessica Lachs (01:04:47):
Yeah.
Lenny Rachitsky (01:04:49):
Is there a clever name for this chatbot per chance?
Jessica Lachs (01:04:51):
So it's not clever. It's called Ask Data AI, and that's named for our internal Slack channel that used to be the open Q&A for people to ask data. So it's not at all clever.
Lenny Rachitsky (01:04:51):
But it's clear.
Jessica Lachs (01:05:07):
But again, it goes with the theme of very, very specific naming conventions that we have at DoorDash; Never Delivered and Ask Data AI.
Lenny Rachitsky (01:05:18):
I love it. Just clarity above all else. That's something I've learned from an editor that I work with. Jess, is there anything else that you want to share or leave listeners with? For folks that are trying to build their data teams, make their data teams more efficient, is there any final wisdom nugget you'd want to share?
Jessica Lachs (01:05:39):
I think the only thing that I want to reiterate is that you don't necessarily need a formal training in whatever it is you're building. And I think that also goes towards the folks that you hire onto the team. And so I mentioned earlier that we've had a lot of folks go to product or go to ops from the team. What I didn't mention is how many folks we've actually had join the analytics team from partner teams. So whether that was from engineering or from our ops team or marketing or finance, we are a net importer of talent as opposed to a net exporter of talent. And I think that that's because my own experience coming over from operations, from being a GM and making that transition into analytics, I find that I'm drawn to other folks who want to make a similar transition.
(01:06:39):
Now again, you have to have the technical skills, and most of these folks have acquired these skills on the job, whatever job they are doing at DoorDash before they transition to the analytics team, or they had maybe some formal training in school. But I love seeing the folks that make that transition and actually want to join the analytics team, even if they're not a career or data scientist. I think it creates a really unique environment where you have folks on the team from different backgrounds with different expertise who can teach each other things. So I can teach you how to build a discounted cash flow model in Excel, and I can learn how to make kick-ass slides from someone who has a background in consulting. And I can learn about common gotchas in statistics from someone who comes to us with a Master's or a PhD in statistics, and we've got our econometrics folks and we've got our economists. We just have a group of people with different backgrounds who can all teach each other how to be better. And we're not all carbon copies of each other.
Lenny Rachitsky (01:07:55):
What I'm hearing is you try to optimize almost for a lot of different complementary skills and very different backgrounds almost.
Jessica Lachs (01:08:02):
Exactly. And also people who have experience at different size companies. I think I love folks from startups who have that hustle and grit, but I also love folks who've seen what scale looks like and can help us see around corners as far as what problems we will encounter as the business is growing. And I think it is not just about a diversity of skill and a diversity of background, it's also diversity of prior company and stage. That can be really a unique way to think about structuring your team so that you get the best of both worlds.
Lenny Rachitsky (01:08:40):
Amazing. Well, just when you thought we were done, we reached our very exciting lightning round. Are you ready?
Jessica Lachs (01:08:47):
I am. Let's do it.
Lenny Rachitsky (01:08:48):
Let's do it. Okay. First question, what are two or three books that you've recommended most to other people?
Jessica Lachs (01:08:55):
I tend to read fiction, particularly historical fiction, and I love spy novels. So I think my brain is always in problem-solving mode even when reading. A recent book that I read that I enjoyed was The Rose Code by Kate Quinn, and it's about women code breakers in World War II, and I really enjoyed that. But rather than recommending a book... I guess I did just recommend a book, but rather than recommending another book, I am going to recommend the Libby app and supporting your local public library because I love the library and I love Libby, so I'll give that as my other recommendation.
Lenny Rachitsky (01:09:37):
Beautiful. Very on brand with sharing economy, company stuff. Libby. Cool. Okay, next question. Favorite recent movie or TV show?
Jessica Lachs (01:09:46):
Yeah, another one. I don't actually watch a lot of TV, definitely don't watch a lot of movies. In fact, haven't seen some of the movie greats. I get yelled at a lot by my friend. "I can't believe you haven't seen that." I tend to re-watch things, so series from the past, over and over again. I think it's just like how I shut my brain off. So I've recently re-watched The West Wing, which is one of my favorite shows of all time, probably for the 50th time.
Lenny Rachitsky (01:10:15):
Oh my God.
Jessica Lachs (01:10:16):
And Alias, which was a Jennifer Garner series from the early 2000s. Also, Spy. So I'm noticing a theme. I think I really love the spy genre. But yeah, I've watched those. They're both great, but not at all current.
Lenny Rachitsky (01:10:33):
Perfect. Perfectly acceptable. Do you have a favorite product that you recently discovered that you really love?
Jessica Lachs (01:10:39):
This is a bit of a curveball. So Korean sunscreens. So I burn really easily, so I have to wear sunscreen and I love Korean sunscreens. I was introduced to them by a friend of mine, and they're just far superior to what we have in the US. So I highly recommend people give Korean sunscreens a try, particularly there's a Beauty of Joseon on branded sunscreen. It's just amazing and is delightful to wear, which is important when you have to wear it every day.
Lenny Rachitsky (01:11:09):
I've been trying to wear more sunscreen as I age, and so this is a really good tip. Was that a brand you recommended?
Jessica Lachs (01:11:15):
Yeah. So Beauty of Joseon is the brand.
Lenny Rachitsky (01:11:15):
Beauty of Joseon.
Jessica Lachs (01:11:18):
There's another brand, Isntree, which also has a great sunscreen. But I'll be honest, almost every Korean sunscreen I've tried is great.
Lenny Rachitsky (01:11:28):
Okay. I'm Googling this as soon as we get off. Do you have a favorite life motto that you often come back to and share and or share with family and friends even more [inaudible 01:11:39]?
Jessica Lachs (01:11:40):
I do. So there's a John Steinbeck quote, which I'm not big on quotes, but I like this one, which is that, "It's a common experience that a problem difficult at night is resolved in the morning after the committee of sleep has worked on it." I find that that's something I really live by. First off, I love sleep and I try to get as much of it as possible. But the other thing is that if I'm stuck on a problem or if I am writing a response to something like a tense issue or an emotional issue, often I find that if I put down my thoughts, go to sleep, check it in the morning, I end up with a better outcome. So all of a sudden you have a new perspective and clarity on a problem you were stuck on, or you realize that you weren't clear in the way you were communicating your thoughts because you were emotional about something and you're able to put together a much better response to an e-mail or to whatever problem you're handling. So sleep can solve lots of problems.
Lenny Rachitsky (01:12:46):
I love sleep as well. I'm always telling my wife, "Let's go to sleep." Like, "Okay, I'll be there soon." I love that advice. Okay, two more questions. Who's influenced you most in your career? Is there someone that comes to mind?
Jessica Lachs (01:13:00):
So I think two answers, a multi-part answer. So I think first my career has been in male-dominated industries and I've worked with just some incredible women who've really influenced me. When I was a banker, there were two senior bankers, Vanessa Roberts and Gina Tarone at Lehman Brothers where I worked. And they were just so incredible. They were just so good at their jobs and I found that really inspiring.
(01:13:29):
And then at DoorDash, Tia Sherringham, who is our GC, and Liz Jarvis-Shean, who leads comms, are just dominant in their fields. And I think that that's really empowering and have been big influences on me to just see strong, powerful women kicking ass and that helps me believe that I can do the same. So that's one answer.
(01:13:54):
And then the other answer, sort of cliche, but my parents. My mom was a statistician at the UN before she got married, and she actually chose to stay home and raise three children, so I'm the youngest. And when I was in, I think it was elementary school, decided to go back to school, switch careers and become a nurse. And so the fact that she embarked on this completely new career in her forties after 15 years as a stay-at-home mom and my father supported this. I think that that was really, really influential and was probably the first time I saw that you can do whatever you put your mind to, no matter your age, no matter your circumstances. So that was really influential and I don't think I've ever told her that. So hi, Mom.
Lenny Rachitsky (01:14:44):
Hi Mom. Thank you, mom.
Jessica Lachs (01:14:45):
Yeah, I think that was influential for my career. Definitely.
Lenny Rachitsky (01:14:49):
That's a beautiful answer. Fun fact, I worked with Liz at Airbnb. Your person you just mentioned in the comms team.
Jessica Lachs (01:14:57):
[inaudible 01:14:57]. She is great.
Lenny Rachitsky (01:14:58):
She's amazing. Final question. So when you joined DoorDash, imagine it wasn't obvious that it was going to work. I imagine it was still like, "This was a crazy idea. Maybe it'll work, maybe not." Is there a moment you recall where you're like, "I think this is going to be a big success? I think this is actually going to work out?"
Jessica Lachs (01:15:14):
To be honest, I went into DoorDash because I wanted to learn for the experience. I thought it was interesting, problems with interesting people. I never thought too much about whether it would work. I of course wanted it to work and was very competitive and wanted to win. I think there's two moments that stand out. One was when the third party market share data showed that we had become the number one player after, I think we started at number four or five. And I think that that was really exciting to see the trajectory and to see us gain in category share. That was exciting. I probably didn't see it until months after it had happened because we don't spend a ton of time focusing on it, but I do remember somebody wanted to include the graph in some presentation, some sales material, and we're like, "Oh, we're number one. That's incredible. We used to be number five." So I'd say that that was one.
(01:16:17):
The other one that stands out was, the first talk I gave in a lot of these startup talks in the early days in Boston, and I'd asked the audience, "How many of you have used DoorDash?" And there'd be like three people who would raise their hand. And then it was a few years ago, maybe 2018, 2019, and I was giving a talk and I asked the audience, "How many of you have used DoorDash?" And almost everyone's hands went up. And that was actually pretty memorable for me because in my mind, we were still the small startup that no one had heard of where I had to over enunciate the D's in DoorDash. So people didn't think I worked for Jordash, the nineties' denim company. And so that was pretty meaningful to me when just so many people had used the product or were consumers of DoorDash. It was pretty exciting. And I still get excited. I saw DoorDash mentioned in a book recently that I was reading. It was like, "We're in the book." So those little things when you become part of the cultural lingo that I think are really, really special.
Lenny Rachitsky (01:17:33):
Well, I'm a very happy customer of DoorDash. I've never had a Never Deliver. It's always there, sometimes a little late. Usually it's perfect. Thank you for everything you do. Go team DoorDash.
(01:17:44):
Two final questions. Where can folks find you online if they want to follow stuff that you do? I know you've been doing more writing on LinkedIn and things like that, so just help people understand where to find you and how can listeners be useful to you?
Jessica Lachs (01:17:55):
Yeah, so as you mentioned, to find me LinkedIn, I don't have a huge social presence, but I am on LinkedIn and I am currently writing a series of blog posts about my experience building a global analytics org at DoorDash. Some of the lessons I've learned over the last 10 years. So definitely check those out.
(01:18:16):
And as far as your second question of how listeners can be useful to me, I guess read the post on LinkedIn and I'd love to hear what people think, whether you agree with my point of view or not. That being said, be nice. I want honest feedback, but I want kindness as well. So yeah, just engage with the content and let me know what y'all think. I think I do have a broader ask, which is just to encourage folks listening to TruthSeek, something I take seriously at DoorDash. It's a company value. But there's a lot of misinformation out there and it's often up to us as individuals to figure out what's fact and what's fiction. So I have a plea for folks to do your best, to search for the truth and speak the truth, and I think we'll all be better off for it. And of course, use DoorDash.
Lenny Rachitsky (01:19:13):
Of course.
Jessica Lachs (01:19:14):
Yes, there are three things that listeners can do.
Lenny Rachitsky (01:19:18):
You're at DoorDash.com. That was awesome. I love that last point as well in addition, to use DoorDash. Jessica, thank you so much for being here.
Jessica Lachs (01:19:27):
Thank you for having me. It was a lot of fun.
Lenny Rachitsky (01:19:29):
Same for me. Bye, everyone.
(01:19:32):
Thank you so much for listening. If you found this valuable, you can subscribe to the show on Apple Podcast, Spotify, or your favorite podcast app. Also, please consider giving us a rating or leaving a review as that really helps other listeners find the podcast. You can find all past episodes or learn more about the show at lennyspodcast.com. See you in the next episode.